The Comprehensive Guide to the Data Annotation Process
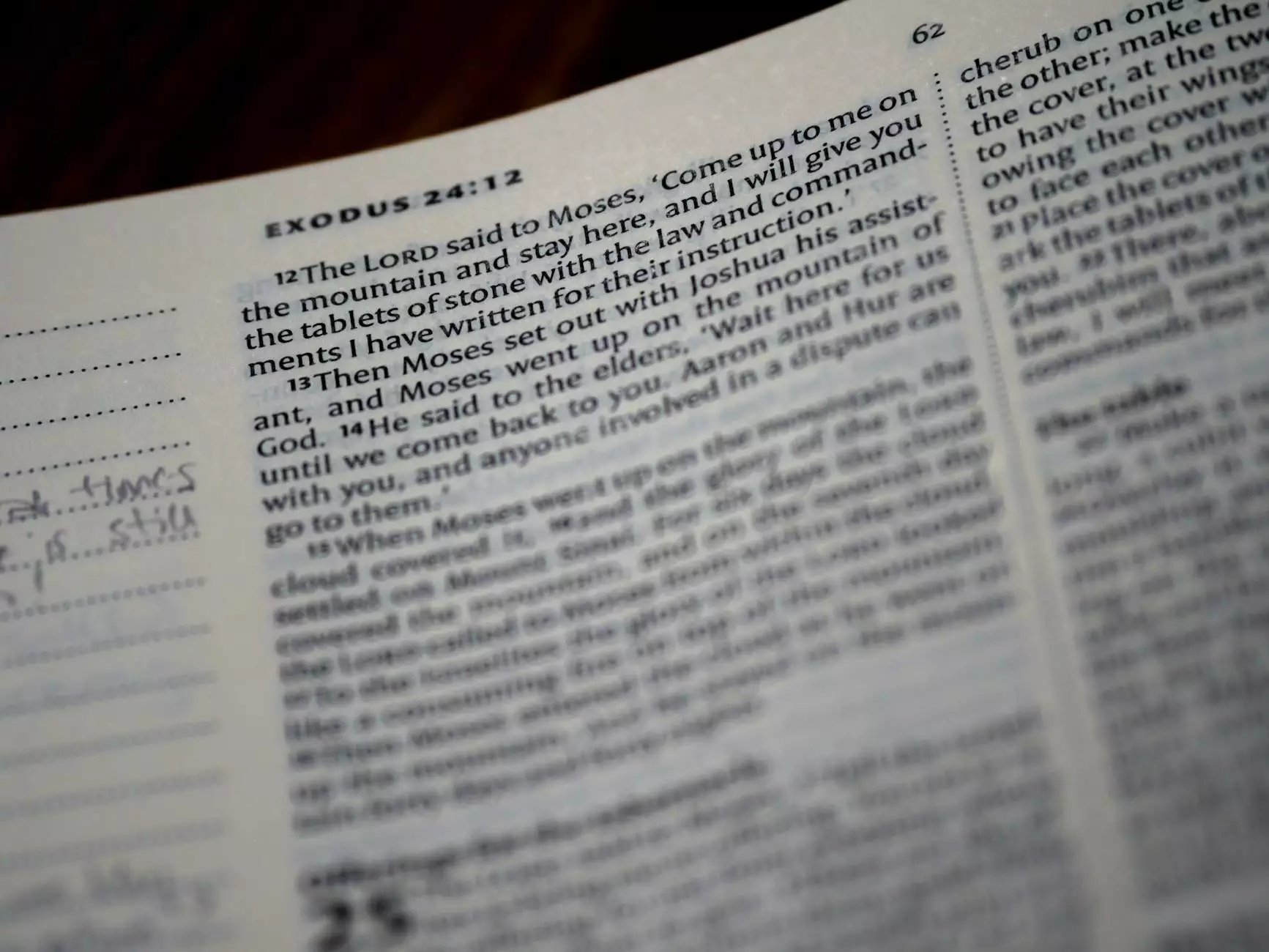
The data annotation process plays a critical role in the advancement of artificial intelligence (AI) and machine learning (ML) technologies. As businesses, especially in sectors like Home Services, Keys & Locksmiths, increasingly adopt these technologies, understanding and implementing effective data annotation strategies becomes essential. This guide delves deep into the data annotation process, its significance, methodologies, and applications across various industries.
What is Data Annotation?
Data annotation is the process of labeling and tagging data to make it understandable for AI and ML systems. This process helps build accurate models that can interpret patterns, recognize objects, understand natural language, and perform various tasks based on the data they are trained on.
The Importance of the Data Annotation Process
In today's fast-paced digital world, the data annotation process is crucial for various reasons:
- Improves Data Quality: Accurate annotation enhances the quality of data used for training AI models, leading to better performance.
- Enables Better Decision Making: Businesses can leverage annotated data to make informed decisions based on insights derived from AI models.
- Enhances User Experience: Improved AI systems provide better services, thereby enriching the overall customer experience.
- Competitiveness: Companies that utilize data annotation effectively can stay ahead of competitors by offering innovative solutions.
The Data Annotation Process Explained
The data annotation process can be broken down into several key steps:
1. Data Collection
The initial stage involves gathering data from various sources. This data can be in the form of images, videos, text, or audio. It's essential to ensure that the collected data is relevant to the project goals.
2. Selection of Annotation Type
Based on the nature of the data and the desired outcome, businesses must choose the appropriate type of annotation. Common types include:
- Image Annotation: Labeling objects within images for computer vision tasks.
- Text Annotation: Tagging parts of text, such as entities and sentiments, useful for natural language processing.
- Video Annotation: Identifying and labeling actions or objects within video frames.
- Audio Annotation: Labeling segments of audio for speech recognition tasks.
3. Annotation Guidelines Development
To ensure consistency and accuracy, businesses should create clear annotation guidelines that annotators must follow. This includes defining categories, labeling rules, and the overall objectives of the project.
4. Annotator Selection
Choosing the right annotators is vital. They can be in-house teams or outsourced professionals. It's essential that annotators are trained and equipped to understand the specific requirements of the project.
5. Annotation Execution
This step involves the actual data labeling. Annotators review the data according to the predefined guidelines and add the required labels. It’s crucial to monitor this phase to maintain quality.
6. Quality Assurance
Quality assurance is a pivotal part of the data annotation process. It involves reviewing the annotated data to identify errors and inconsistencies, ensuring that the final dataset meets the required standards.
7. Feedback and Iteration
The feedback loop is essential for continual improvement. Annotators should receive feedback on their work, and adjustments should be made to the guidelines and processes based on this feedback to enhance overall quality.
8. Delivery of Annotated Data
After thorough quality checks, the final annotated dataset is delivered to the clients or integrated into the respective machine learning models for training.
Applications of Data Annotation in Different Businesses
The data annotation process is extensively used in various business sectors, each benefiting uniquely from high-quality labeled data:
Home Services
In the home services industry, businesses often utilize data annotation to enhance customer service through AI-driven platforms. For instance:
- Chatbots: Annotated conversational data helps train chatbots to provide accurate responses, improving customer interaction.
- Image Recognition: Annotated images of various home repair functionalities allow apps to suggest the right services based on customer queries.
Keys & Locksmiths
For businesses in the keys and locksmiths sector, data annotation can play a significant role in security and operational efficiency:
- Video Surveillance: Annotated video footage improves the accuracy of crime detection systems.
- Customer Sentiment Analysis: Text annotation aids in understanding customer feedback on services, helping businesses improve their offerings.
Choosing the Right Data Annotation Service
When selecting a data annotation service, consider the following factors:
- Experience and Reputation: Investigate the service provider's background and success stories in your industry.
- Accuracy and Quality Control: Ensure they have a robust quality assurance process in place.
- Scalability: Choose a service that can scale with your needs as your data volumes grow.
- Data Security: Ensure they adhere to strict data privacy and security measures to protect sensitive information.
Challenges in Data Annotation and How to Overcome Them
While the data annotation process is highly beneficial, it does come with its challenges:
1. High Volume of Data
Managing and annotating large volumes of data can be overwhelming. To tackle this, businesses should consider segmenting data into manageable chunks and implementing effective project management tools.
2. Quality Consistency
Maintaining consistent annotation quality can be difficult, especially with multiple annotators. Providing comprehensive training and establishing robust guidelines can mitigate this risk.
3. Cost Considerations
Outsourcing data annotation can become expensive. To control costs, companies can utilize semi-automated annotation tools that combine human efforts with machine learning assistance.
Conclusion
The data annotation process is a pivotal element in the development and deployment of effective AI and ML systems. By investing time and resources into understanding and optimizing this process, businesses, particularly in the Home Services and Keys & Locksmiths sectors, can significantly enhance their operational efficiency, customer satisfaction, and competitive edge.
As the need for high-quality, annotated data continues to grow, organizations should prioritize developing comprehensive data annotation strategies to ensure their success in an increasingly AI-driven marketplace. By doing so, they are not just investing in technology, but in the future of their business.