Transform Your Data Annotation Process with an Innovative Image Labeling Tool
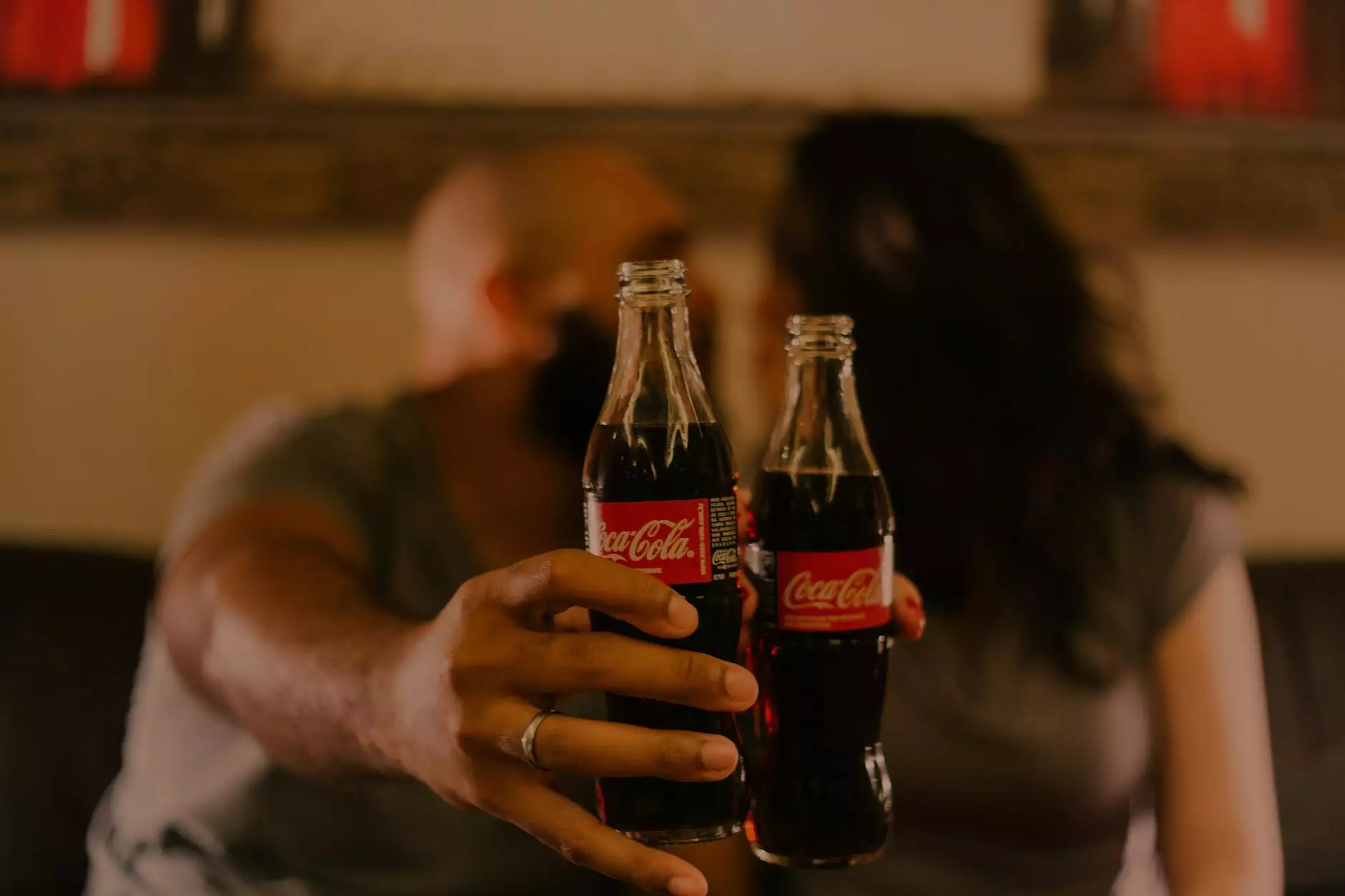
The world of data annotation has rapidly evolved, thanks in part to the surge in artificial intelligence and machine learning technologies. With image labeling tools becoming essential, businesses like KeyLabs.ai are at the forefront, offering sophisticated platforms to enhance your data handling capabilities. In this article, we will explore how an image labeling tool can revolutionize your data annotation process, ensuring accuracy, efficiency, and high-quality results.
Understanding the Significance of Data Annotation
Data annotation is a critical process wherein raw data is labeled to enhance its usability for machine learning models. As businesses collect vast amounts of data, the need for accurate and efficient annotation methods becomes paramount. This reliance on high-quality annotated data drives the demand for advanced image labeling tools.
What is an Image Labeling Tool?
An image labeling tool is a software solution designed to assist in tagging images with relevant information. This process often involves:
- Bounding Box Creation: Drawing boxes around objects within images to identify and classify them.
- Semantic Segmentation: Assigning labels to each pixel, enhancing detail in image classification.
- Object Tracking: Following the movement of objects across a series of images or frames.
Why Invest in an Image Labeling Tool?
When businesses invest in an image labeling tool, they unlock several benefits that can streamline operations and improve model performance.
1. Enhanced Accuracy
Accuracy is key in data annotation. Utilizing an image labeling tool significantly reduces human error. The tool’s advanced algorithms help maintain consistency and precision in labeling, resulting in higher quality datasets for machine learning models.
2. Increased Efficiency
Manual data annotation can be labor-intensive and time-consuming. By implementing an image labeling tool, organizations can automate many aspects of the annotation process, dramatically increasing productivity. Less time spent on tedious tasks means faster turnaround times for projects.
3. Scalability
As businesses grow, so too do their data needs. An effective image labeling tool can scale with your business, accommodating larger volumes of data without compromising on quality. This scalability ensures that you remain competitive in an increasingly data-driven market.
Key Features to Look For in an Image Labeling Tool
When selecting an image labeling tool, there are several key features to consider:
1. User-Friendly Interface
A user-friendly interface is essential for ensuring that your team can effectively use the tool without extensive training. Look for solutions that offer intuitive navigation and easy access to features.
2. Customization Options
The ability to customize the labeling process allows teams to tailor the tool to their specific project needs. Whether you need polygonal segmentation or unique labels, flexibility is vital.
3. Collaboration Capabilities
In many businesses, data annotation is a collaborative effort. A good image labeling tool should facilitate teamwork, allowing multiple users to annotate data simultaneously and track changes effortlessly.
4. Integration with Other Tools
Seamless integration with existing workflows and tools can save time and effort. Ensure that the image labeling tool you choose can work harmoniously with other software solutions your team already uses.
5. Support for Various File Formats
Your image labeling tool should support a range of image formats (such as JPEG, PNG, TIFF, etc.), providing you with greater flexibility in your data projects.
How KeyLabs.ai Supports your Data Annotation Needs
At KeyLabs.ai, we offer a robust image labeling tool that encompasses all the essential features mentioned above while leveraging state-of-the-art technology. Here’s how our platform stands out:
1. Advanced Annotation Features
Our image labeling tool empowers users with advanced annotation capabilities, including semantic segmentation, bounding box creation, and polygon labeling, ensuring comprehensive coverage of data requirements.
2. AI-Driven Automation
We utilize AI algorithms to automate repetitive tasks, allowing users to focus on more complex labeling challenges. This not only enhances efficiency but also maintains high accuracy levels across large datasets.
3. Real-Time Collaboration
Our platform supports real-time collaboration, enabling teams to work together effectively, share feedback instantly, and monitor progress on annotation tasks.
Case Studies: Success Through Effective Image Labeling
Many organizations have successfully utilized our image labeling tool to improve their data annotation processes. Here are a few case studies highlighting their achievements:
1. Case Study: E-commerce Retailer
An e-commerce retailer employed our image labeling tool to annotate product images for better categorization and searchability on their site. By quickly labeling thousands of images, they improved their product discoverability by 35%, leading to a substantial increase in sales.
2. Case Study: Autonomous Vehicle Company
A company developing autonomous vehicle technology used our tool to label images for object recognition. By integrating our platform into their workflow, they reduced their data annotation time by 50%, which expedited the development of their self-driving algorithms.
Challenges in Image Labeling and How to Overcome Them
While image labeling tools offer numerous benefits, there are challenges in implementing them effectively. Here are common challenges and solutions:
1. High Variability in Data
Different images can vary significantly in quality, lighting, and content. This variability can make annotation inconsistent. To overcome this, it's essential to establish clear labeling guidelines and utilize tools that adapt to these variations.
2. Ensuring Labeling Consistency
Inconsistent labeling from different annotators can lead to inaccurate datasets. Utilizing an image labeling tool with built-in quality checks and a comprehensive review process can ensure consistency across all data.
3. Training Data Scarcity
A lack of sufficient training data can hinder the effectiveness of machine learning models. Conducting initial rounds of manual annotation combined with the capabilities of an image labeling tool can help build a robust dataset.
The Future of Image Labeling Tools
The future of image labeling tools is bright, with emerging technologies poised to further enhance their capabilities. Here are a few trends to watch:
1. Machine Learning Integration
As machine learning continues to evolve, the integration of AI algorithms in image labeling tools will only become more sophisticated. This will lead to automated suggestions, minimizing manual input requirements.
2. Increased Focus on Edge Cases
Tools that can accurately label edge cases—images that fall outside normal parameters—will become increasingly valuable, particularly in industries like healthcare where precision is vital.
3. Enhanced User Experience
Future image labeling tools will prioritize user experience, making it easier for teams to collaborate, make annotations, and manage datasets efficiently.
Conclusion
The role of an image labeling tool in today's data-driven landscape cannot be overstated. By leveraging advanced data annotation platforms, businesses can significantly enhance their operational efficiency, leading to improved outcomes and insights. Visit KeyLabs.ai to explore our innovative solutions, designed to meet your data annotation needs and propel your business forward in the digital age.