The Transformative Impact of Semantic Segmentation Video in Data Annotation
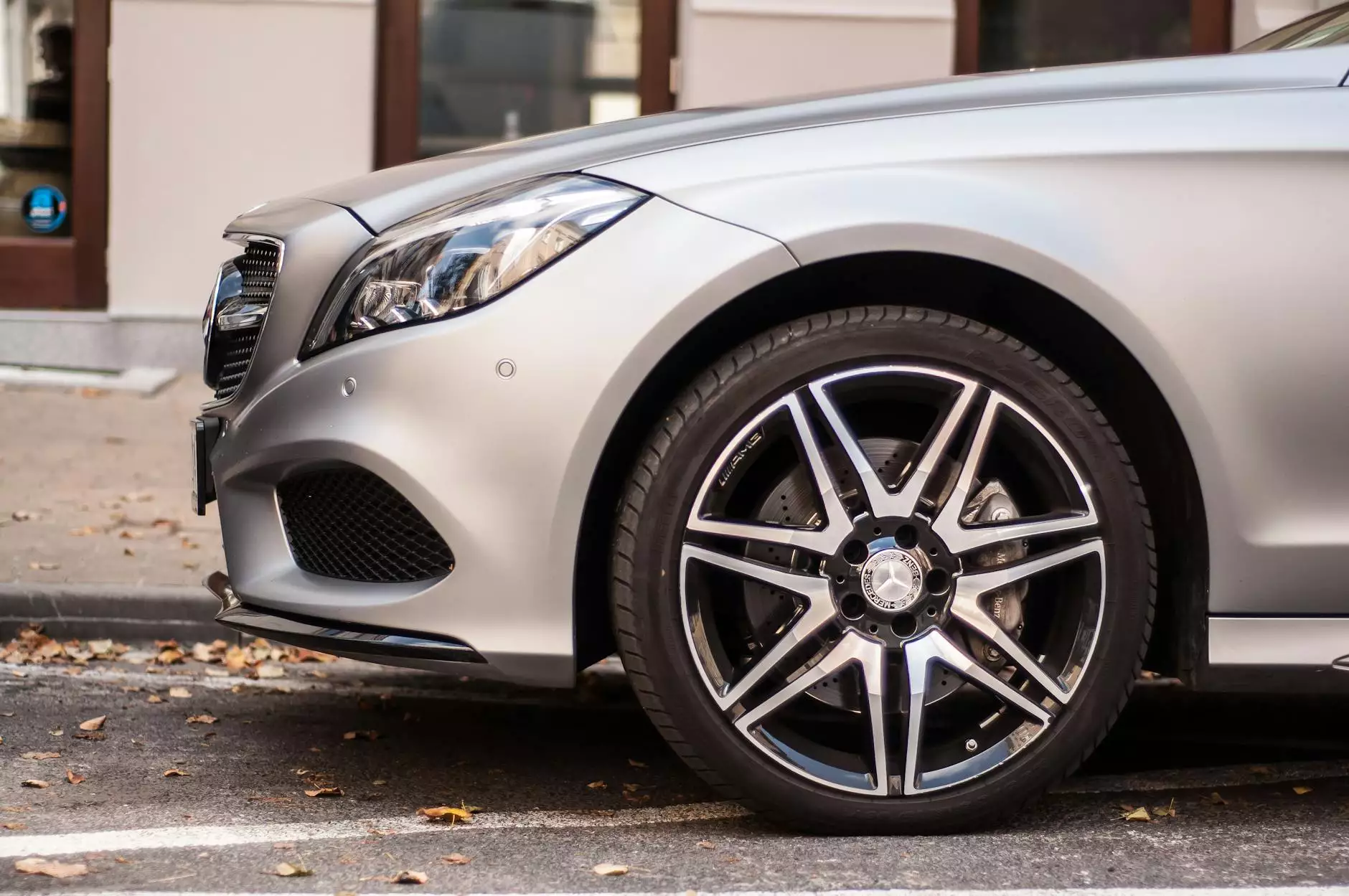
In today's rapidly evolving technological landscape, the need for accurate and efficient data annotation methods has never been greater. Among these methods, semantic segmentation video stands out as a revolutionary approach that enhances the capability of data annotation tools and data annotation platforms. This article comprehensively explores the mechanics, applications, and undeniable advantages of semantic segmentation in video datasets, positioning it as a cornerstone for organizations looking to leverage advanced machine learning and artificial intelligence technologies.
Understanding Semantic Segmentation
Before diving into the specifics of semantic segmentation video, it is essential to grasp the foundational concept of semantic segmentation itself. Semantic segmentation is the process of classifying each pixel in an image or video frame into a category. This means that every pixel is labeled with a specific class, which could correspond to different objects, backgrounds, or contextual elements within the scene.
For example, in an urban driving scenario, a semantic segmentation model would identify pixels corresponding to cars, pedestrians, bicycles, road signs, and other elements crucial for understanding the environment. This granularity significantly enhances the performance of various AI applications, particularly in the fields of robotics, autonomous driving, and video analytics.
The Role of Video in Semantic Segmentation
While semantic segmentation is commonly applied to still images, the dynamic nature of video adds another layer of complexity and opportunity. When we incorporate time as a dimension, semantic segmentation video analyzes continuous frames, allowing algorithms to understand not just static components, but also motion and interactions.
Benefits of Semantic Segmentation Video
- Enhanced Contextual Understanding: By analyzing sequences of frames, semantic segmentation video provides insights into the interactions between objects and their environments over time.
- Real-Time Processing: With advancements in computational power, semantic segmentation can be applied in real-time scenarios, vital for applications such as autonomous vehicles and surveillance systems.
- Improved Object Tracking: Understanding object movement and behavior through video segmentation aids in developing more sophisticated tracking algorithms.
- Dynamic Scene Interpretation: By incorporating temporal data, semantic segmentation can adapt to changes in scene composition, enhancing AI models' robustness.
Applications of Semantic Segmentation Video in Various Industries
The power of semantic segmentation video is harnessed across numerous sectors, demonstrating its versatility and importance.
1. Autonomous Vehicles
In the automotive industry, semantic segmentation video is crucial for enabling vehicles to navigate complex environments. By accurately segmenting road signs, pedestrians, vehicles, and lane dividers in real time, these systems ensure safer and more efficient transportation.
2. Video Surveillance
Security systems leverage semantic segmentation video to automatically detect and classify unusual behavior or events. This capability allows for better situational awareness and quicker response times in security scenarios.
3. Robotics
In robotics, semantic segmentation video enables machines to understand their surroundings better, allowing them to interact intelligently and safely with humans and objects in dynamic environments.
4. Healthcare
In healthcare, video analysis through semantic segmentation can assist in monitoring patient movement and behavior, which is particularly vital in rehabilitation and elderly care settings. Accurate segmentation allows for better tracking and understanding of patient progress.
5. Augmented and Virtual Reality
Augmented (AR) and Virtual Reality (VR) applications benefit immensely from semantic segmentation video as it provides a more immersive experience, enhancing interaction between virtual objects and the real world.
Challenges in Implementing Semantic Segmentation Video
Despite its numerous benefits, the adoption of semantic segmentation video is not without challenges:
- Data Quality: High-quality, accurately annotated training data is paramount for effective model training. Poor annotations can lead to significant errors in segmentation.
- Computational Resources: High-resolution video requires substantial processing power and memory, posing challenges for real-time applications.
- Model Complexity: Developing a robust semantic segmentation model can be complex due to the need for advanced architecture and optimization techniques.
Best Practices for Effective Semantic Segmentation Video
To overcome challenges and ensure effective outcomes, organizations can follow specific best practices when implementing semantic segmentation video:
1. Utilize High-Quality Datasets
Invest in comprehensive datasets that represent diverse scenarios and conditions, ensuring the model encounters a broad spectrum of data during training.
2. Leverage Advanced Annotation Tools
Use cutting-edge data annotation tools that support efficient and accurate semantic segmentation. Platforms like KeyLabs.ai specialize in providing robust annotation solutions, enhancing productivity and accuracy in data preparation.
3. Employ Quality Control Mechanisms
Implement rigorous validation processes to ensure that the segmented data is accurate and meets the required standards. Regular audits can help maintain data integrity.
4. Experiment with Network Architectures
Explore various deep learning architectures that are specifically tailored for video data analysis. Networks such as CNNs (Convolutional Neural Networks) and RNNs (Recurrent Neural Networks) have been shown to perform well in these tasks.
Conclusion: The Future of Semantic Segmentation Video
As we look towards the future, the importance of semantic segmentation video in driving advancements in AI and machine learning cannot be overstated. Organizations that harness this technology, particularly through robust data annotation platforms like KeyLabs.ai, will not only enhance their operational efficiency but also position themselves at the forefront of innovation in their respective fields.
The versatility and applicability of semantic segmentation in video underscore its significance across various industries. As data continues to grow exponentially, the demand for sophisticated methodology will only intensify. Organizations must adapt and invest in these technologies to thrive in an increasingly competitive landscape.
In conclusion, embracing semantic segmentation video is not just about keeping pace with technology; it is about revolutionizing how businesses operate and innovate. The journey towards a smarter, more data-driven world is underway, and those equipped with the right tools and strategies will lead the way.